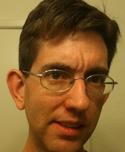
卢克·穆尔豪瑟(Luke Muehlhauser): One interesting feature of your own thinking (Hayworth 2012) about whole brain emulation (WBE) is that you are more concerned with modeling high-level cognitive functions accurately than is e.g.Sandberg (2013)。Whereas Sandberg expects WBE will be achieved by modeling low-level brain function in exact detail (at the level of scale separation, wherever that is), you instead lean heavily on modeling higher-level cognitive processes using a cognitive architecture calledACT-R。Is that because you think this will beeasierthan Sandberg’s approach, or for some other reason?
Kenneth Hayworth:我认为关键的区别是,哲学家are focused on whether mind uploading (a term I prefer to WBE) is possible in principle, and, to a lesser extent, on whether it is of such technical difficulty as to put its achievement off so far into the future that its possibility can be safely ignored for today’s planning. With these motivations, philosophers tend to gravitate toward arguments with the fewest possible assumptions, i.e. modeling low-level brain functions in exact detail.
As a practicing cognitive and neuroscientist I have fundamentally different motivations. From my training, I am already totally convinced that the functioning of the brain can be understood at a fully mechanistic level, with sufficient precision to allow for mind uploading. I just want to work toward making mind uploading happen in reality. To do this I need to start with an understanding of the requirements, not based on the fewest assumptions, but instead based on the field’s current best theories.
To use an analogy, before airplanes were invented one could argue that heavier-than-air flying machines must be possible in principle even if it meant copying a bird’s flapping motions etc. This is a sound philosophical argument but not one that engineers like the Wright Brothers would focus on. Instead they needed to know the crucial elements necessary for heavier-than-air flight –lift, drag, thrust. Understanding these allowed them to start building and testing, refining their theories and engineering.
如果我们想创建技术以将某人的思想上传到计算机模拟中,并且模拟具有与原始的记忆,智力,个性和意识相同的记忆,那么我们就需要从对这些认知功能的顶级理解开始。寻找这些的地方是认知科学领域,以及诸如ACT-R之类的智能行为的高度研究计算模型。亚博体育官网
I have focused my research on understanding how the computational elements of cognitive models such as ACT-R (symbolic representations, production rules, etc.) are likely mapped onto the neural circuits in the brain. This has led me to concrete predictions on exactly what technologies would be necessary to preserve, scan, and simulate a person’s brain. Those are the technologies I am currently working on.
卢克·穆尔豪瑟(Luke Muehlhauser):ACT-R是多么广泛使用或接受?只要浏览文献,它似乎并不是在认知神经科学中特别占主导地位,尽管这也许只是因为不同类的高级模型是主导的。例如。ACT-R在新的1100多页中似乎并未提及Oxford Handbook of Cognitive Neuroscience, nor in the 1800+ pages of theEncyclopedia of Behavioral Neuroscience。我很少见到不是支持者的人提到的ACT-R。
Kenneth Hayworth: Looking at themain website for ACT-R我总共计算了与ACT-R理论或使用ACT-R建模框架有关的1,035个个人出版物。该出版物记录来自数百名研究人员,并可以追溯到几十年来,并在今天持续强劲。亚博体育官网ACT-R不会被认为是“认知神经科学”理论,因为它没有谈论神经元(尽管有尝试将ACT-R映射到我将讨论的神经框架上)。相反,ACT-R在认知科学领域被正确分类。当然,在计算机科学,心理学,神经科学,神经病学,认知科学,人工智能,计算神经科学等领域都存在一个混乱的重叠。ACT-R当然是在考虑这些各个领域的限制下设计的。
根据其智能行为模型的典型抽象水平,最好将认知科学与神经科学区分开。认知科学致力于通过传统的处理模拟和通过算法来处理各种复杂性的符号表示的计算系统。亚博体育苹果app官方下载一般而言,人类智能行为的认知科学模型以代表性和算法形式呈现。例如,在感知实验中,人类注意力和记忆保留效应的模型可能认为我们的思想包含4个缓冲区,可用于存储代表单个字母的符号令牌,这些字母代表该主题的观看屏幕上短暂闪烁。请注意,像这样的认知科学模型并未谈论神经元甚至大脑区域,但它们对行为响应,响应时机,错误率,学习率等进行了具体测试的预测。-效应可以在详细和精确的情况下进行详细和精确测试。心理物理实验。
If you are looking for explanations for how humans understand natural language, or how they solve problems and reason about goals, or, crucially, how the mind creates and maintains a “self model” underlying our consciousness then cognitive science models at this level of abstraction are really the only place to look. Neuroscience should be seen as talking about the implementation details underlying the algorithms and representations assumed by such cognitive science models.
现在,认知科学作为一个领域已经存在了很长时间,并且已经为人类如何执行各种动作产生了各种各样的小型模型和算法假设。Way back in 1973 the great cognitive scientist Allen Newell wrote a paper called “You can’t play 20 questions with nature and win” in which he pointed out to the cognitive science community that it needed to strive for “unified theories of cognition” which were designed to explain not just one experimental result but all of them simultaneously. He proposed a particular computational formalism called a “production system” that could provide the basis for such a unification of different cognitive science models. He and others developed that proposal into the widely used SOAR model of human cognition (see his book “Unified Theories of Cognition” for a complete explanation of production systems as a model of the human mind).
There have been many production system models of the human mind based upon this original work but Anderson’s ACT-R theory is currently the standard-bearer of this class. As such, ACT-R should not really be thought of as just another computational model, instead you should think of ACT-R as attempting a summary of all results from the entire field of cognitive science –a summary in the form of a general computational framework for the human mind. Below is a screen capture of the ACT-R website which keeps a list of all publications and ACT-R models generated over the years. You can see that the categories span the entire field of cognitive science (e.g. language processing, learning, perception, memory, problem solving, decision making, etc.)
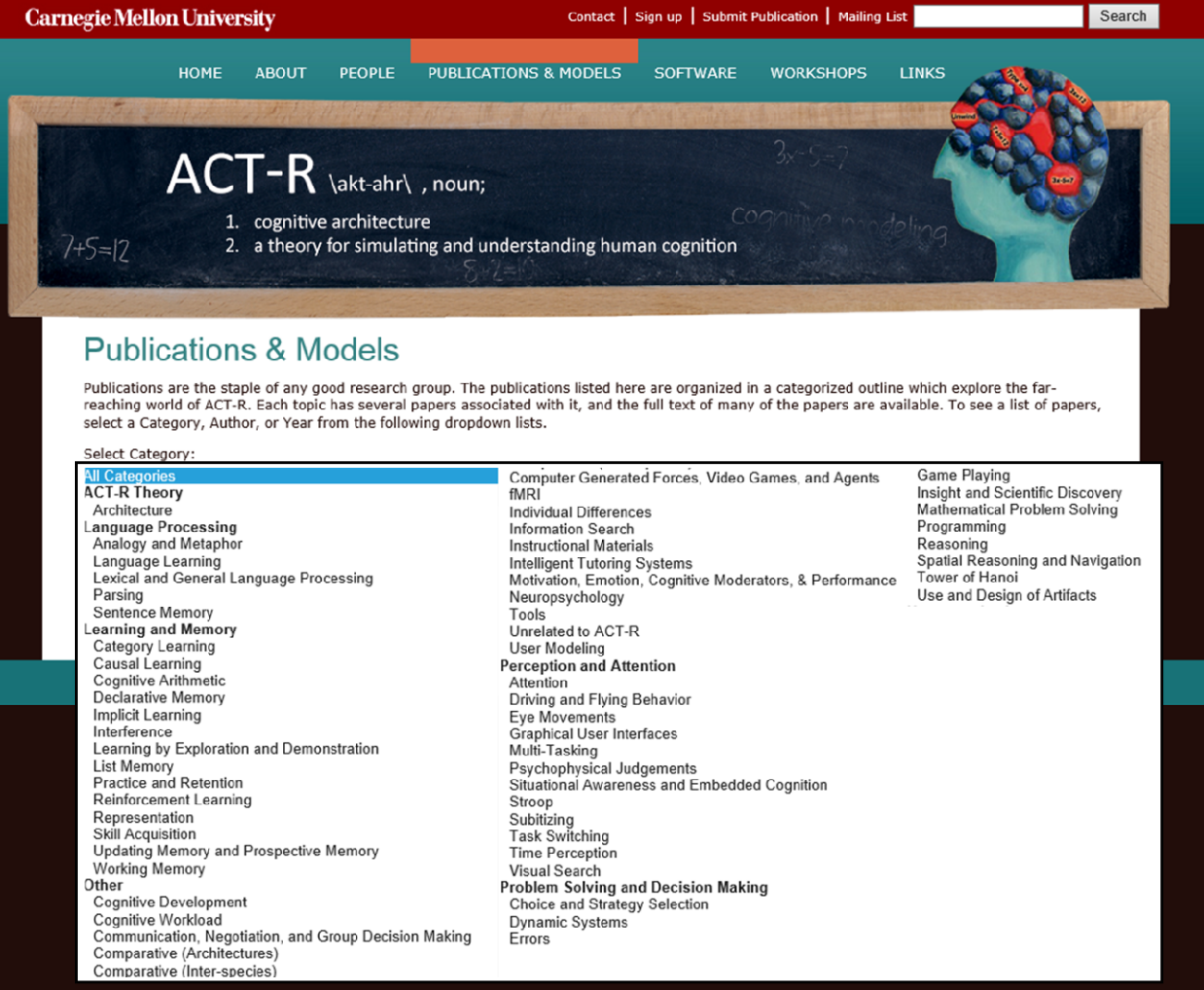
另外,您应该注意,由于ACT-R是一个计算框架,因此在ACT-R框架内构建的模型确实是“工作”的,因为句子解析的ACT-R模型实际上会解析句子。
当您说“ [ACT-R]在认知神经科学中似乎并不特别占主导地位”时,我会听到您的声音。这是绝对正确的。我遇到的大多数神经科学家从未听说过ACT-R或生产系统,通常不熟悉认知科学领域的大多数伟大发现。亚博体育苹果app官方下载这是一个真正的悲剧,因为认知科学和神经科学仅被正确地看作是对同一器官 - 大脑的两个级别描述。没有认知科学的抽象(符号,声明性记忆块,生产规则,目标缓冲区等),神经科学面临着无法克服的差距,以解释神经元如何引起我的想法。这就像必须通过描述其在晶体管级别的操作来解释计算机程序如何工作。只是无法完成。为了创建一个可以理解的Microsoft Word理论,必须引入中间级别的抽象级别(内存缓冲区,如果是循环等)。当涉及到大脑如何产生思维时,情况也是如此。
I argue that any complete theory of how the mind is generated by the physical brain must include at least the following four levels of description:
- 意识和自我的哲学理论(例如:托马斯·梅辛格(Thomas Metzinger)的书“无人:自我模型的主观性理论”)
- Cognitive science models of the human cognitive control architecture (Example: John Anderson’s book “How can the human mind occur in the physical universe” –his most recent overview of ACT-R)
- 摘要海马记忆系统,感知特征层次结构,强化学习模式识别网络等的摘要“人工”神经网络体系结构。亚博体育苹果app官方下载
- Electrical and molecular models of real biological neurons and systems (Example: Kandel, Schwartz and Jessell’s book “Principles of Neural Science”)
如果您不能在这些描述级别之间进行巧妙的切换,了解每个级别的核心原理以及它们之间的构建方式,那么您就没有准备好了解完成整个大脑仿真所需的内容。简单地了解神经科学是不够的。
一个今天在这存在的最薄弱环节s hierarchy of descriptive levels is the link between #2 and #3. This is why I have been putting considerable effort into showing how the symbolic computations assumed by ACT-R might be implemented by standard artificial neural network models of autoassocative memory etc. My 2012 publication“Dynamically partitionable autoassociative networks as a solution to the neural binding problem” is a first attempt at this. I am currently preparing a new publication and set of neural models designed to make this link even clearer. My goal is to demonstrate to the neuroscience community that the level of abstraction assumed by cognitive science models like ACT-R is not so removed from the neural network models which neuroscientists currently embrace. An effective bridging of this gap between cognitive science models and neural network models has the potential to release a wave of synergy between the fields, in which the top-down constraints of cognitive science can directly inform neuroscience models, and the bottom-up constraints of neuroscience can directly inform cognitive models.
Luke:ACT-R的指定性如何?特别是,您能举例说明ACT-R做出一个令人惊讶的新颖定量预测,在实验之后,事实证明是正确的?(即使尚未发生这种情况,ACT-R仍然可能有用,但是如果有这样的例子,那么很高兴知道它们!)
Kenneth:回答这个问题的困难是,ACT-R实际上是创建大脑功能模型的“框架”,它本身并不是模型。研究亚博体育官网人员通常使用ACT-R框架来创建特定认知任务的模型 - Say建模学生如何学习解决代数表达式。为了成功,该模型必须对错误率和错误类型,响应速度以及该速度随练习等的增加等定量预测。任何特定的ACT-R模型都包含一组初始的“生产”(符号)模式匹配如果是当时的规则)和“声明性记忆块”(符号记忆),这些(符号记忆)被认为已被个人学到了。该模型将使用这些初始生产规则和内存块产生智能行为。它还将改变规则和块的权重,因为它通过反复试验学习,并且在与模拟环境相互作用时会产生新的生产规则和新的记忆。
Now one can ask your question about novel predictions regarding either a particular ACT-R model or regarding the ACT-R framework itself. To pick one particularly good example of an ACT-R model making “surprising and novel” predictions you might want to look at the paper: “A Central Circuit of the Mind” (Anderson, Fincham, Qin, and Stocco 2008). In that paper (and a host of follow up papers) an ACT-R model of a cognitive task was used to predict not only behavioral responses but fMRI BOLD activation levels and timing in subjects performing the cognitive task. Considering that the ACT-R community spent many years developing models of brain function without fMRI data as a constraint, it is particularly intriguing to see how well those models have fared when viewed against this new type of data.
As for predictions of the ACT-R architecture itself, I would like to point to perhaps ACT-R’s central prediction going straight back to its inception –the prediction that there are two types of learning: “procedural” and “declarative”. This distinction is so well established now (think of studies of the amnesia patient HM) that it is hard to remember that this was anything but settled when ACT-R was invented. In fact, this is the key difference between the ACT-R and the SOAR cognitive architectures. SOAR was built on an incorrect prediction –that all knowledge in the brain was encoded as procedural rules. This incorrect prediction is why SOAR lost favor with the cognitive science community and why ACT-R is still widely used.
You ask “How well-specified is ACT-R?”. This is a good question. The ACT-R architecture has changed significantly over the years as new information has become available. Over the last decade it went through a dramatic simplification in the complexity that was allowed for the pattern matching in production rules. It also has set the values of some of its core performance and learning parameters (for example the time it takes to execute a single production rule is now set to be 50ms). These ACT-R architectural parameters were of course determined by fitting data to behavioral experiments, but since ACT-R is meant to be a theory of the brain as a whole, individual models are not allowed to arbitrarily change these parameters to fit their particular data. In effect, these ACT-R architectural features have become a significant constraint on the degrees of freedom modelers have when proposing new ACT-R models of particular cognitive tasks. The success or failure of these tighter specifications of the ACT-R framework parameters are best judged by how successful it still is for modeling a wide range of behaviors. As for that I would again point to the wide range of publications using ACT-R.
I don’t think one should judge ACT-R on the basis of novel predictions however. It is designed as a summary theory to account for a large body of cognitive science facts. It should, and has, changed when its predictions were found unsound. For example, ACT-R’s original model of production matching was essentially found untenable given what we know about neural networks, so it was jettisoned for a new simpler version.
我认为判断RACT-R的最佳方法是了解它的意思和不意味着什么。ACT-R用于在计算级别,而不是在神经实现级别上对认知任务进行建模。它在计算层面上是非常具体的,并且已被证明可以为在此级别上为广泛的智能人类行为提供令人满意的(暂定)解释。我认为,这是我们在这个计算层面上的最佳人物模型,因此我们作为神经科学家应该将其用作理解大脑神经回路的高级计算类型的起点。
Luke:回到WBE。您是导致FHI的2007年研讨会的参与者Whole Brain Emulation: A Roadmap报告。您是否介意就报告中的一些关键问题分享自己的估计?特别是,该报告的表4(第39页)总结了可能的建模并发症,以及估计WBE必要的可能性以及实施的难度。您最强烈地不同意这些估计中的哪个估计?(“ WBE”是指报告所谓的成功标准6a(“社会角色拟合仿真”),以便搁置意识和个人身份问题。)
Kenneth: First I would like to say how fantastic it was for Anders and the FHI to put on that workshop. It is amazing to see how far the field has progressed in the meantime. I agree with most of the main conclusions of that report, but since you asked for what I disagree with I will focus in on those few points on which I have reservations.
该报告以标题为“对全系统理解的几乎不需要”的部分开始,作者指出:“ WBE的一个重要假设是,为了模仿大脑,我们不需要理解整个系统,而是我们亚博体育苹果app官方下载只是我们只是需要一个数据库,其中包含有关大脑的所有必要的低级信息以及对本地更新规则的了解,以改变大脑状态。”(第8页)
I agree that it is technically possible to achieve WBE without an overall understanding of how the brain works, but I seriously doubt this is the way it will happen. I believe our neuroscience models, and the experimental techniques to test them, will continue their rapid advance over the next few decades so that we will reach a very deep understanding of the brain’s functioning at all levels well before we have the technology for applying WBE to a human. Under that scenario we will understand exactly which features listed in table 4 of the report are needed, and which are unnecessary.
作为一个具体的示例(本来是有争议的),有几种类型的皮质神经元被认为可以为皮质的主要兴奋性细胞提供一般的饲料和反馈抑制。这些抑制细胞有效地调节了在给定时间活跃的兴奋性细胞的百分比(即神经代表的“稀疏”)。我怀疑这些抑制性细胞的细节是否将在将来完全建立,因为它在计算机模型中会更容易,更可靠,以实施直接发射的这种稀疏性。现在,这一建议可能会吸引您的许多读者作为一个特别冒险的捷径,他们可能会问:“我们如何确定这些抑制性神经元的详细功能对于思维的运作并不重要?”情况可能是这种情况,但是我的观点是,到我们有了真正尝试人类的技术时,我们将确定什么“快捷方式”是可以接受的。检验这些假设所需的实验比WBE本身要简单得多。
Luke:在您看来,如果资助资金,可以在未来10年中向WBE显示出很大的进步,那么哪些特定的,下一个研究项目是什么?亚博体育官网
Kenneth: Over the next ten years progress in WBE is likely to be tied to progress in the field of connectomics (automated electron microscopic (EM) mapping of brain tissue to reveal the precise connectivity between neurons). Since the 2004 invention of theserial block face scanning electron microscope(SBFSEM, or SBEM) there has been an explosion of interest in this area. SBFSEM was the first automated device to really show how one could, in principle, “deconstruct brain tissue” and extract its precise wiring diagram. Since then the SBFSEM has undergone dramatic improvements and has been joined by several other automated electron microscopy tools for mapping brain tissue at the nanometer scale (TEMCA,atum-sem,fib-sem)。凯文·布里格曼(Kevin Briggman)和戴维·博克(Davi Bock)(该领域的两个领导人)写了很棒的review articlein 2012 covering the current state of all of these connectomics imaging technologies. In short, neuroscientists finally have some automated tools which can image the complete synaptic connectivity of the neural circuits they have been studying for years by behavioral and electrophysiological means. Several “high profile” connectomics publications have recently come out (Nature2011,Nature2011,Nature2013,Nature2013,Nature2014)提供这样的工具可以实现的目标,但是这些出版物仅代表了可能是神经科学的革命的“冰山一角”,从长远来看,这场革命将导致WBE。
There is, however, one thing that is currently holding back the entire field of connectomics – the lack of an automated solution to tracing neural connectivity. Even though there has been fantastic progress in automating 3D EM imaging, each of these connectomics publications required a small army of dedicated human tracers to supplement and “error-correct” the output of today’s inadequate software tracing algorithms. This roadblock has been widely recognized by connectomics practitioners:
“迄今为止,[A] LL细胞分辨率连接组学研究涉及数千至数千小时的[人工劳动神经]重建。尽管成像速度大大提高,但重建速度却大大落后。对于任何提议的密集电路重建(小鼠新皮层[柱],嗅球,鱼脑和人类新皮层[列]…),分析时间估计值至少是一个比已完成的数量级的数量级至少一个数量级迄今为止……这些设想的项目的要求大约为每个项目的手动劳动数十万小时。这些巨大的数字构成了细胞连接组中所谓的分析差距:尽管成像较大的电路变得越来越可行,但重建它们并不是。”- 莫里茨·赫尔姆斯塔德(Moritz Helmstaedter)(自然方法评论2013)
A full solution to this “analysis gap” will likely require advances on three fronts:
- 改进的3D成像分辨率
- 改善组织保存和染色
- More advanced algorithms
当今的大多数连接成像技术仍然依赖于组织的物理切片,该组织实际上将EM成像的“ Z”分辨率限制为20nm。但是,那fib-sem相反,技术使用聚焦的离子束在每个EM成像步骤之间可靠地“抛光” <5nm的组织层。这意味着FIB-SEM数据集可以用体素分辨率为低至5x5x5nm的组织成像组织。当试图遵循肾小管神经元过程时,这种额外的分辨率至关重要,该过程通常可以收缩至直径<40nm。这种改进的FIB-SEM分辨率已经demonstrated大大增加户田拓夫的有效性y’s tracing algorithms. I have been working on extending this FIB-SEM technique to make it capable of handling arbitrarily large volumes. I do this by using a heated, oil-lubricated diamond knife to losslessly subdivide a large block of tissue into chunks optimally-sized (~20 microns thick) for high-resolution, parallel FIBSEM imaging. I will have a paper on this coming out later this year.
This higher resolution (FIB-SEM) imaging, along with likely advances in tracing algorithms, should finally be able to achieve the goal of fully-automated tracing of neural tissue –i.e. it should overcome the “analysis gap”, eliminating the reliance on human tracing.
也就是说,只要组织优化研究ved and stained for EM imaging. Unfortunately, today’s best tissue preservation and staining protocols are limited to <200 micron thick tissue slabs. This volume limit also represents a fundamental roadblock to connectomics, and there is only one researcher that I know of that has seriously taken up the challenge to remove this limitation –肖恩·米库拉(Shawn Mikula)。他做了实质性进展达到这个目标,但仍有大量工作。
因此,关于您有关“特定的,可访问的下一个研究项目”的问题,我将提供以下内容:开发一个计划,以保存,染色和无效的整个亚博体育官网鼠标大脑,以进行随机访问fib-sem的成像,以及a project to build a “farm” of hundreds of inexpensive FIB-SEM machines capable of imaging neural circuits spanning large regions of this mouse’s brain.
I believe that the Mikula protocol can be extended to provide excellent EM staining for all parts of a mouse brain (Mikula, personal communications). I also believe, with sufficient work and funding, that this mouse protocol could be made compatible with my “lossless subdivision” technique which would allow the entire mouse brain to be quickly and reliably chopped up into little cubes (~50x50x50 microns in size) any of which could be loaded into a FIB-SEM machine for imaging. I also believe, with sufficient funding, that today’s generation of expensive (>1million USD) FIB-SEM machines could be redesigned, and drastically simplified for mass-manufacture.
我认为这样一个项目确实可以在十年的时间内进行处理,实际上,它将为神经科学家提供一种工具(我称之为“它”连接组天文台”) to map out the neural circuits involved in vision, memory, motor control, reinforcement learning, etc. all within the same mouse brain. Allowing researchers to see not only an individual region’s circuits, but also the long distance connections among these regions which allow them to act as a coherent whole controlling the mouse’s behavior. I think it is at this level that we will begin to see rudiments of the types of coordinated, symbol-level operations predicted by cognitive models like ACT-R, the types of executive control circuits which in us evolved to underlie our unique intelligence and consciousness.
We must remember and accept that the long-term goal of human whole brain emulation is almost unimaginably difficult. It is ludicrous to expect significant progress toward that goal in the next decade or two. But I believe we should not shrink away from saying that that goal is the one we are ultimately pursuing. I believe neuroscientists and cognitive scientists should proudly embrace the idea that their fields will eventually succeed in revealing a complete and satisfying mechanistic explanation of the human mind and consciousness, and that this understanding will inevitably lead to the technology of mind uploading. There is so much hard work to be done that we cannot afford to neglect the tremendous payoffs awaiting in the event we succeed.
Luke: Thanks, Kenneth!
Did you like this post?You may enjoy our other对话posts, including: